AI helps you optimize robot energy consumption by integrating advanced sensors for real-time monitoring, enabling efficiency algorithms that minimize waste, and predicting demand with advanced models. It adjusts operations dynamically using real-time data, schedules smart charging, and incorporates renewable energy sources for sustainability. These combined strategies ensure your robots use energy more efficiently and reliably. Keep exploring how these innovations work together to boost your systems’ performance even further.
Key Takeaways
- AI analyzes real-time sensor data to dynamically adjust robot operations and reduce unnecessary energy use.
- Machine learning models predict optimal energy consumption patterns based on historical and environmental data.
- AI-driven algorithms optimize trajectory planning and task scheduling for maximum energy efficiency.
- Intelligent power management systems enable instant adjustments to energy distribution based on current demands.
- Integration of renewable energy sources with AI forecasting ensures sustainable and cost-effective robot operation.
Leveraging Advanced Sensors for Precise Energy Monitoring
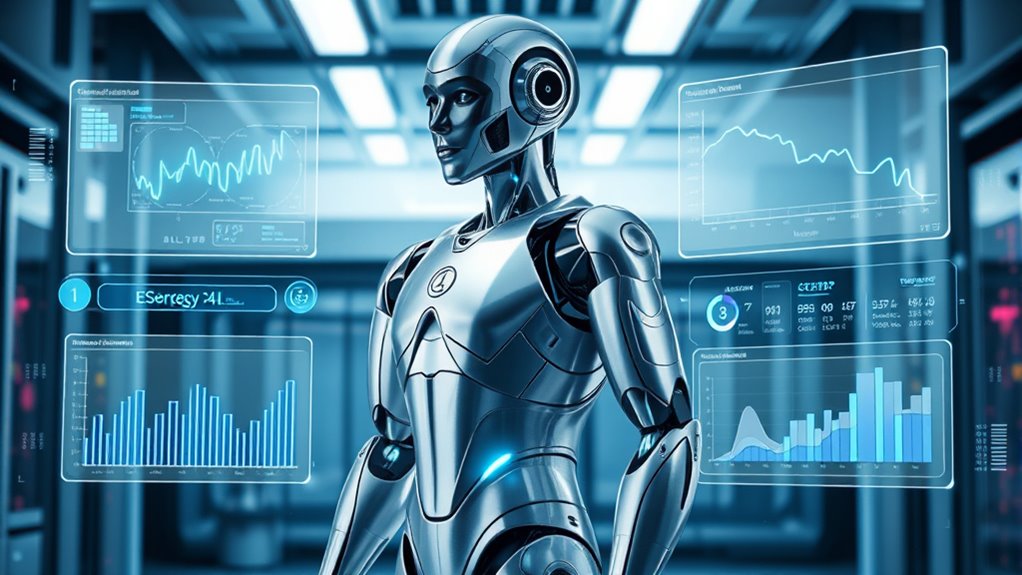
Leveraging advanced sensors is essential for achieving precise energy monitoring in robots. By integrating LiDAR and RADAR sensors, you can detect obstacles and navigate efficiently, reducing unnecessary energy use.
Integrating LiDAR and RADAR sensors enhances obstacle detection and energy-efficient navigation in robots.
An integrated sensor network combines data from multiple sources, providing real-time insights that improve operational awareness. Environmental sensors monitor conditions like temperature and humidity, allowing robots to adjust their functions for ideal energy efficiency. Incorporating sensor calibration techniques further enhances data accuracy, ensuring optimal performance.
Haptic sensors enable delicate handling, preventing wasteful movements. These sensors deliver immediate feedback to control systems, facilitating dynamic energy adjustments based on current demands. Sensor maintenance is also critical to sustain sensor accuracy over time, minimizing errors in energy consumption.
High-quality fiber optic sensors and RF sensors further refine monitoring, ensuring accuracy. When calibrated correctly, these sensors feed data into AI systems, enabling predictive insights that help you maximize energy consumption proactively.
Additionally, insights from AI in entertainment are increasingly being applied to optimize robotic systems, enhancing their efficiency through smarter data analysis.
Implementing Efficiency Algorithms to Minimize Waste
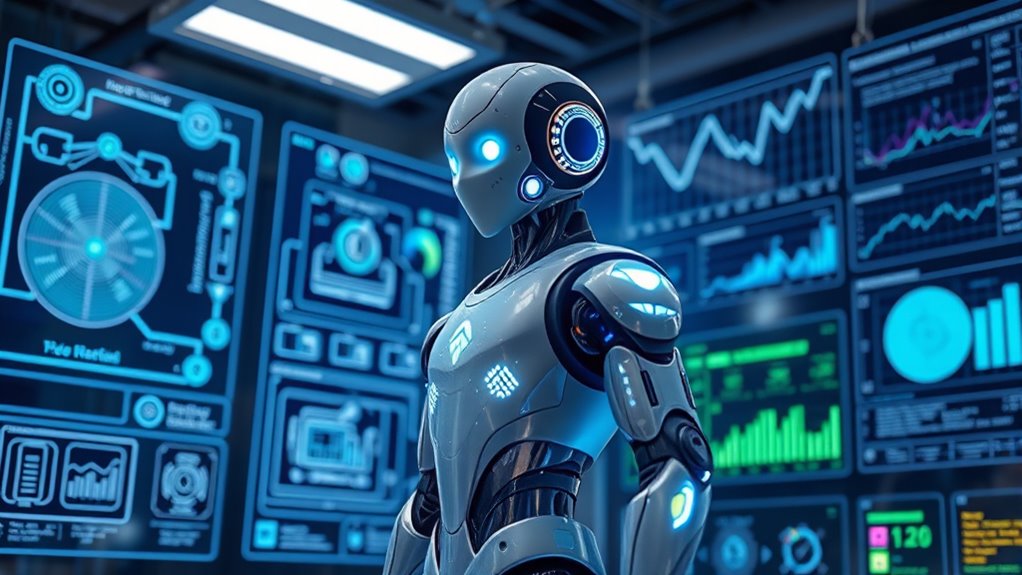
Implementing efficiency algorithms is vital to reducing energy waste in robotic systems. You can use trajectory optimization to find movement paths that use the least energy while completing tasks effectively. Additionally, research indicates that optimizing for AI vulnerabilities can improve safety and reliability in automated systems. Incorporating energy-efficient scheduling strategies can further enhance overall power management. Joint configuration optimization helps identify the least energy-intensive setups by evaluating multiple options. Incorporating energy efficiency metrics—like acceleration, friction, and gravity—ensures movements are optimized for minimal energy use. Balancing performance with energy savings allows your robot to perform tasks quickly without unnecessary waste. Real-time data integration plays a fundamental role, as it enables dynamic adjustments based on sensor feedback, preventing energy from being wasted on inefficient motions. Additionally, understanding the power consumption of different components can guide design choices to improve overall energy efficiency. Moreover, integrating training in SQA principles can help ensure that the software controlling energy management functions reliably and effectively. Recognizing the importance of a creative approach to problem-solving can lead to innovative solutions that further reduce energy consumption in complex systems.
Utilizing Predictive Models for Demand Forecasting

Utilizing predictive models for demand forecasting involves analyzing large volumes of historical sales data to identify patterns and trends. You’ll incorporate past sales figures, seasonal variations, and external economic indicators to refine predictions. You can also consider Hyundai Tuning techniques, which optimize vehicle performance, as an analogy for fine-tuning forecasting models to achieve better accuracy. Knowing store hours can help tailor forecasts to specific shopping times and customer behaviors. Additionally, understanding model calibration techniques can improve the alignment of predictions with actual outcomes, ensuring more reliable forecasts. By continuously monitoring market trends, you can adjust forecasts to stay aligned with real-world changes. Machine learning models trained on extensive datasets predict future customer behavior and market shifts, improving over time through feedback. AI-driven analytics reveal complex, non-linear relationships in data, boosting forecast accuracy. These models also simulate various market scenarios, helping you prepare for demand fluctuations. Incorporating data validation techniques ensures the reliability of the predictions and minimizes errors. Implementing real-time data updates allows forecasts to adapt swiftly to sudden market changes, enhancing responsiveness. Integrating AI with human insights ensures forecasts remain relevant and practical. This approach enhances supply chain efficiency, reduces waste, and optimizes energy use in robots, supporting smarter resource allocation.
Real-Time Adjustments With Dynamic Controls
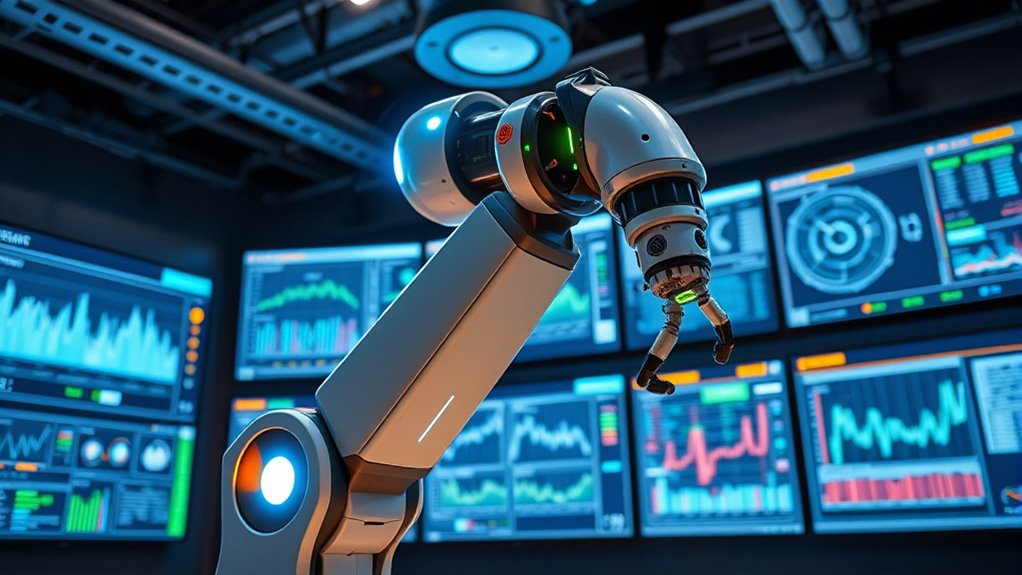
Real-time adjustments with dynamic controls enable robots to adapt their energy consumption instantly based on current conditions. A new sentence with power management and the rest of the sentence. AI-driven analytics interpret real-time energy monitoring data to spot inefficiencies and predict future usage. This feedback allows the system to make immediate adjustments, such as scaling down or shutting off unnecessary components during low-demand periods. AI integrates with dynamic control systems to tweak robot settings on the fly, optimizing energy use without sacrificing productivity. These controls can respond to external factors like weather or utility prices, ensuring energy is used efficiently. Incorporating Gold IRA strategies can also enhance sustainability efforts by aligning energy consumption with eco-friendly investments. Additionally, understanding energy efficiency principles helps refine control strategies for better performance. Implementing automated adjustments based on real-time data further improves overall energy conservation. Continuous AI refinement of control strategies guarantees maximum efficiency. By dynamically managing energy consumption, your operations stay lean, cost-effective, and environmentally friendly—all while maintaining high performance.
Optimizing Charging Schedules Through Smart Power Management
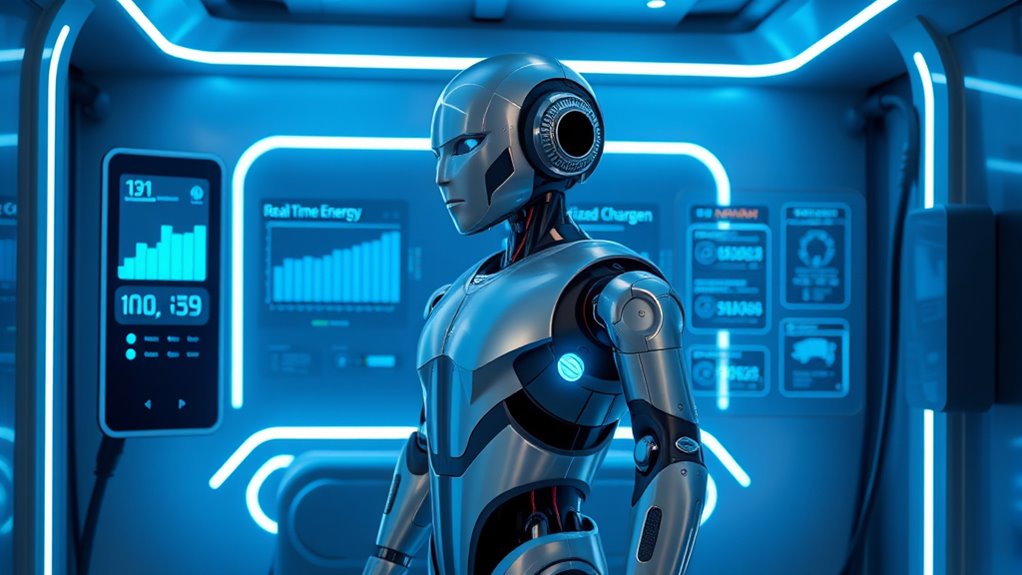
Smart power management leverages AI-driven algorithms to create ideal charging schedules for robots and electric vehicles, ensuring energy is used efficiently and costs are minimized. By analyzing factors like arrival and departure times, energy demand, electricity prices, and charging rate limits, these algorithms optimize when and how devices recharge. Predictive analytics forecast energy usage and user behavior, allowing schedules to adapt proactively. Incorporating dynamic energy management and integrating these systems with IoT enables seamless operation across connected environments, reducing resource waste. Implementing real-time monitoring allows for immediate adjustments to charging activities, further enhancing efficiency. Incorporating energy consumption data can help refine scheduling accuracy and improve overall system performance. Additionally, employing energy-efficient algorithms can significantly optimize power distribution, leading to further reductions in energy waste. Scalable algorithms handle large fleets of robots or vehicles, while Linear Programming models minimize resource consumption. Incorporating celebrity lifestyle insights can inspire innovative approaches to user-centric energy solutions. This approach enhances operational efficiency, decreases energy costs, and supports stable power grid demands—driving sustainable, cost-effective automation.
Integrating Renewable Energy Sources for Sustainable Operations
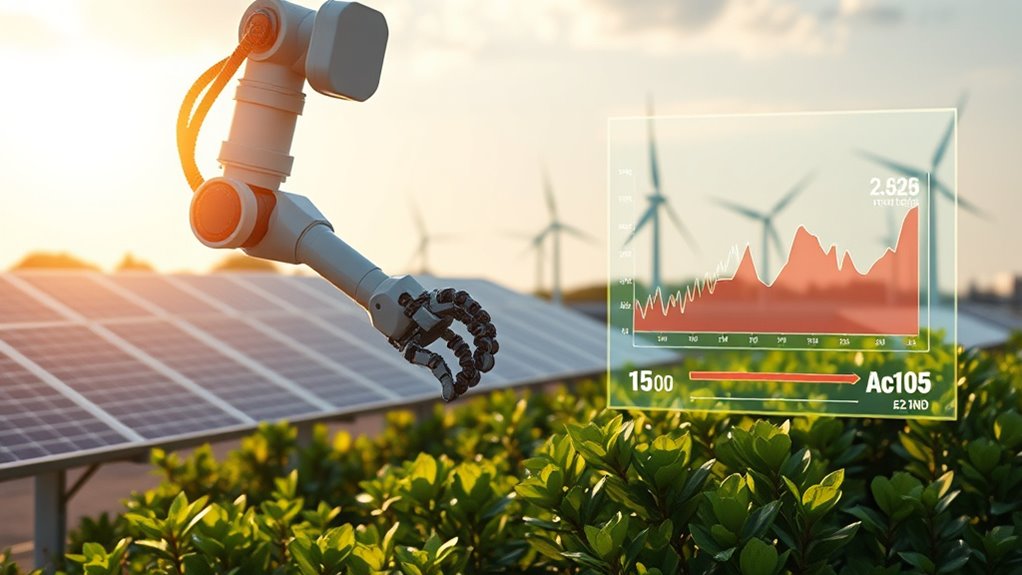
Building on AI-driven power management strategies, integrating renewable energy sources offers a path toward more sustainable and cost-efficient robot operations. As renewable energy grows to supply 35% of global power by 2025, your robots can benefit from cleaner, cheaper energy.
Robotics play a crucial role in reducing installation and maintenance costs for solar panels, wind turbines, and hydroelectric plants, especially in remote locations. They can optimize solar panel cleaning, replace wind turbine blades efficiently, and monitor hydroelectric systems for continuous operation. Nail Tips By leveraging AI, your robots can operate with a reduced carbon footprint, minimize environmental impact, and improve safety by handling hazardous tasks. Incorporating energy-efficient components can further optimize overall system performance. Additionally, advancements in renewable energy integration can help ensure stable energy supply and enhance system reliability.
Integrating renewable energy not only enhances sustainability but also increases operational efficiency and lowers long-term costs for your energy-dependent robotic systems.
Frequently Asked Questions
How Does AI Prioritize Energy Efficiency Across Multiple Robotic Tasks Simultaneously?
You want to know how AI manages multiple robotic tasks for energy efficiency. It does this by using task scheduling to prioritize high-energy tasks during low-demand times, forecasting energy availability, and grouping similar tasks.
Real-time monitoring helps adjust priorities on the fly, while dynamic resource allocation guarantees prime use.
Path optimization, sensor data, and idle modes further reduce energy waste, making your robots operate effectively across all tasks.
What Role Does Machine Learning Play in Adapting Energy Strategies Over Time?
They say “practice makes perfect,” and in AI, that means continuously learning. Machine learning plays an essential role by analyzing past data, adjusting strategies, and improving energy management over time.
You’ll see robots refine their actions through trial and error, balancing exploration and exploitation. This adaptive process ensures they become more efficient, making smarter decisions in real-time and reducing waste, just like a seasoned athlete perfecting their craft.
How Can AI Predict and Prevent Energy-Related System Failures in Robots?
You want to know how AI predicts and prevents energy-related system failures in robots. AI analyzes sensor data to spot patterns and anomalies that signal potential issues.
It then generates real-time alerts and suggests preventive actions, such as targeted maintenance or adjusting energy use. By forecasting energy demands and optimizing control, AI helps you avoid failures, reduce energy waste, and keep your robotic systems running smoothly and efficiently.
In What Ways Can AI Improve the Lifespan of Robot Batteries Through Energy Management?
You might be surprised to learn AI can extend robot battery lifespan by up to 40%. It does this by analyzing usage patterns, predicting battery health, and optimizing charging cycles in real-time.
You can implement smart charging systems, choose high-quality batteries, and reduce idle power to maximize longevity.
AI’s ability to monitor and adapt guarantees your robot batteries last longer, lowering costs and improving overall efficiency.
How Does AI Balance Energy Consumption With Robot Performance Requirements?
In your current question, you want to know how AI balances energy use with robot performance. AI achieves this by analyzing real-time sensor data to adjust movements dynamically, ensuring efficient energy use without sacrificing task quality.
It employs optimization algorithms and learning methods to find the best trade-off between minimal energy consumption and meeting performance goals, allowing your robot to operate smoothly, adapt to changing conditions, and conserve power effectively.
Conclusion
By harnessing AI to optimize robot energy use, you can substantially boost efficiency and sustainability. Advanced sensors, smart algorithms, and predictive models work together to cut waste and adapt in real-time. Charging schedules and renewable integrations further enhance performance. Isn’t it time you embraced AI-driven energy solutions to keep your robots running smarter and greener? The future of robotics is one where energy efficiency isn’t just an option—it’s a necessity.